Subscribe for updates on Pitt NLP Seminar
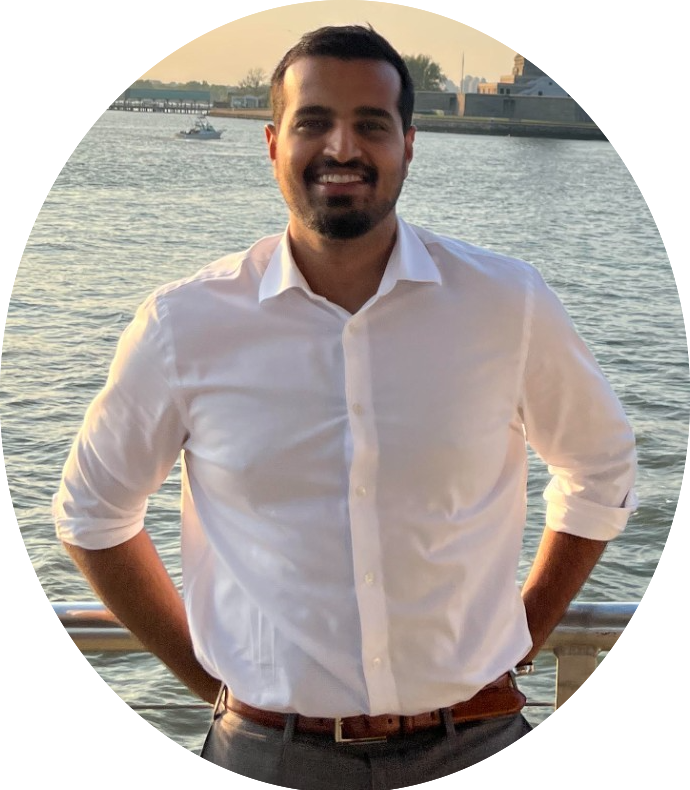
Akshat Gupta
UC Berkeley
Time: 03/12/2025, 4:30 - 5:30 PM (EST)
Bio: Akshat Gupta is a second-year CS PhD student at UC Berkeley, affiliated with the Berkeley Artificial Intelligence Research (BAIR) Lab and the Berkeley Speech Group. His research focuses on knowledge editing and interpretability.
Title: The Past, Present and Future of Knowledge Editing
Abstract: Knowledge editing in large language models enables targeted updates of specific factual information without extensive retraining. In this talk, we discuss existing knowledge editing methods and the current research landscape in knowledge editing which has highlighted its limits at scale. This talk will also talk about ideas that allow scaling existing knowledge editing methods to many thousands of edits. Finally, we conclude by highlighting future directions and existing challenges in the field.
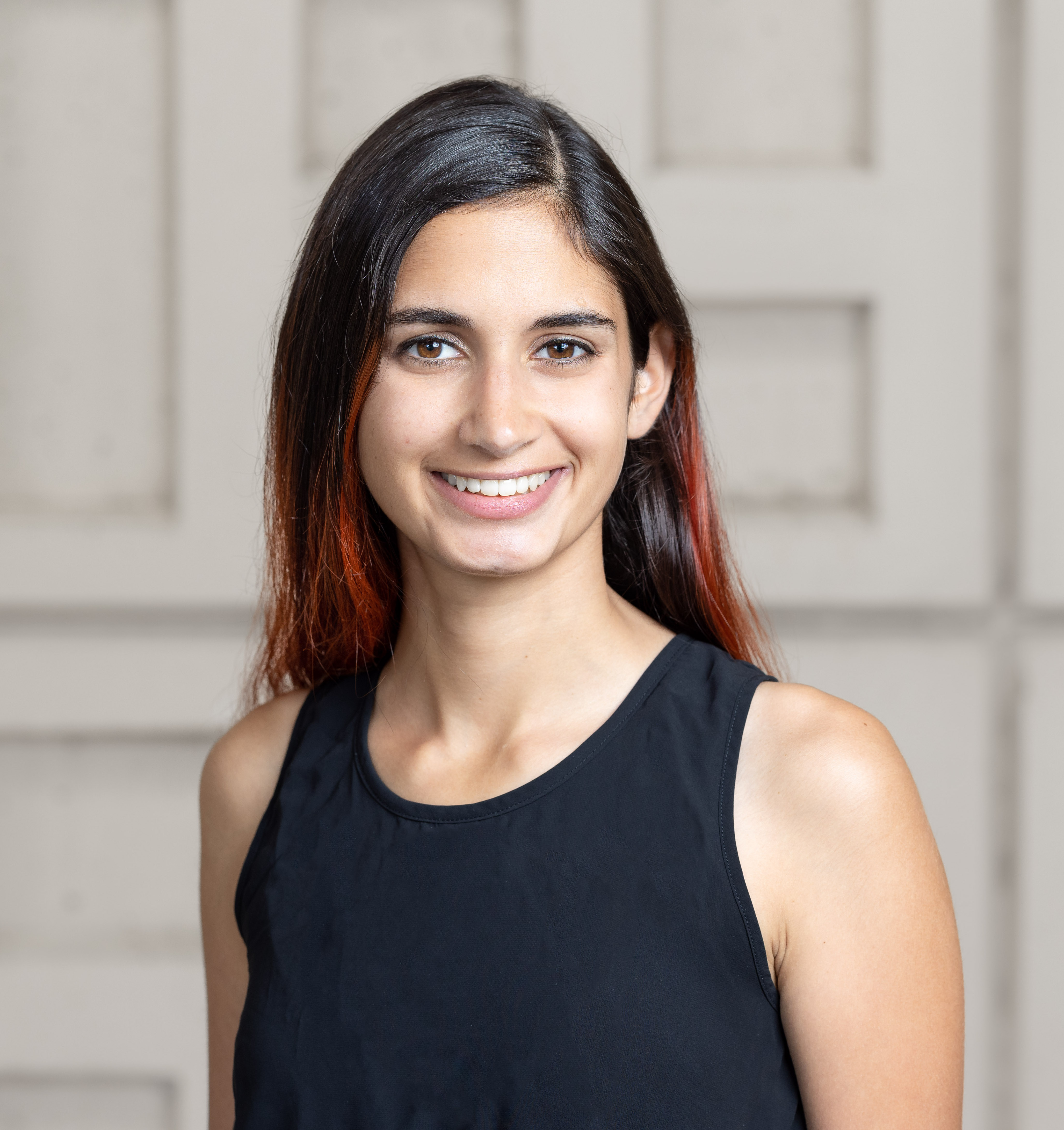
Anjalie Field
Johns Hopkins University
Time: 03/19/2025, 4:30 - 5:30 PM (EST)
Place: In-person (SQ 5317)
Bio: Anjalie Field is an Assistant Professor in the Computer Science Department at Johns Hopkins University. She is also affiliated with the Center for Language and Speech Processing (CLSP) and the new Data Science and AI Institute. Her research focuses on the ethics and social science aspects of natural language processing, which includes developing models to address societal issues like discrimination and propaganda, as well as critically assessing and improving ethics in AI pipelines. Her work has been published in NLP and interdisciplinary venues, like ACL and PNAS, and in 2024 she was named an AI2050 Early Career Fellow by Schmidt Futures. Prior to joining JHU, she was a postdoctoral researcher at Stanford, and she completed her PhD at the Language Technologies Institute at Carnegie Mellon University.
Title: Fairness and Privacy in High-Stakes NLP
Abstract: Practitioners are increasingly using algorithmic tools in high-stakes settings, like healthcare, social services, policing, and education with particular recent interest in natural language processing (NLP). These domains raise a number of challenges, including preserving data privacy, ensuring model reliability, and developing approaches that can mitigate, rather than exacerbate historical bias. In this talk, I will discuss our recent work investigating risks of racial bias in NLP child protective services and ways we aim to better preserve privacy for these types of audits in the future. Time permitting, I will also discuss, our development of speech processing tools for policy body camera footage, which aims to improve police accountability. Both domains involve challenges in working with messy minimally processed data containing sensitive information and domain-specific language. This work emphasizes how NLP has potential to advance social justice goals, like police accountability, but also risks causing direct harm by perpetuating bias and increasing power imbalances.
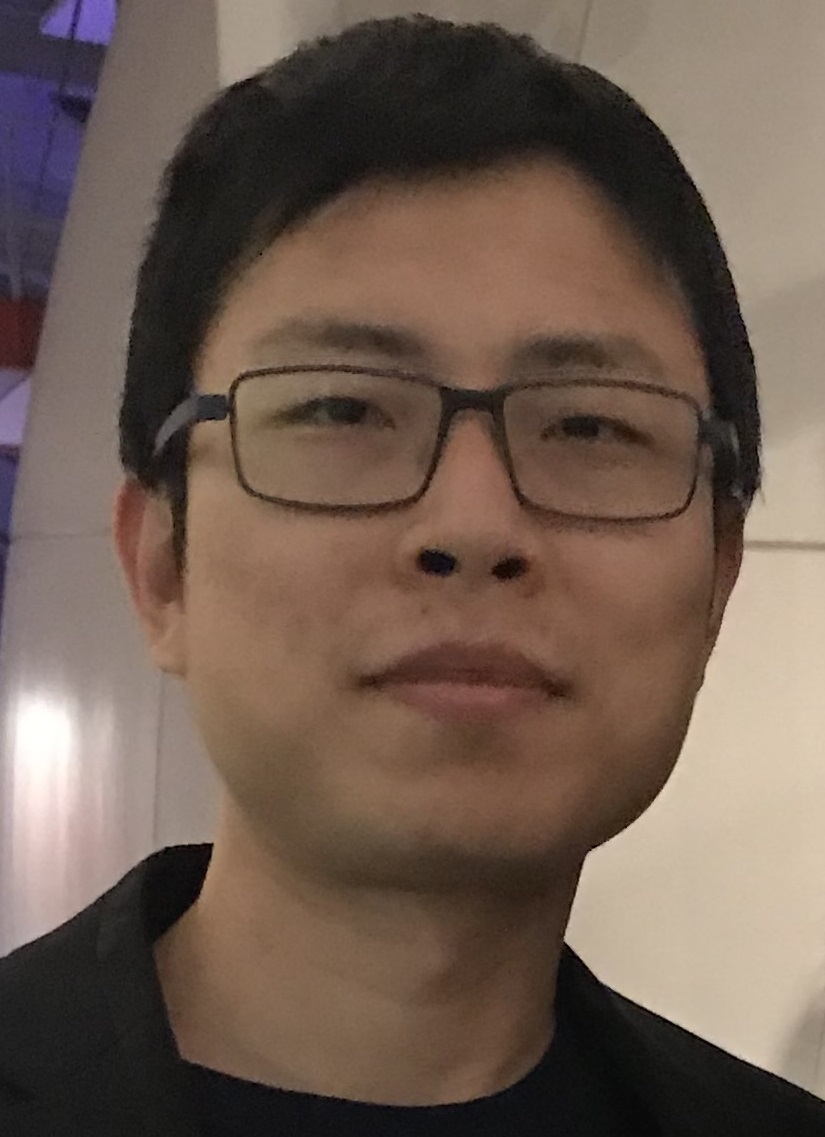
Qifan Wang
MetaAI
Time: 03/26/2025, 4:30 - 5:30 PM (EST)
Place: Zoom
Bio: Qifan is a Research Scientist at Meta AI, leading a team building innovative Deep Learning and Natural Language Processing models for Recommendation System. Before joining Meta, he worked as a Research Engineer at Google Research, focusing on deep domain representations and large-scale object understanding. Qifan received his PhD in computer science from Purdue University in 2015. Prior to that, he obtained both his MS and BS degrees in computer science from Tsinghua University. His research interests include deep learning, natural language processing, information retrieval, data mining, and computer vision. He has co-authored over 100 publications in top-tier conferences and journals, including NeurIPS, ICLR, ICML, ACL, EMNLP, NAACL, CVPR, ICCV, ECCV, SIGKDD, WWW, SIGIR, AAAI, IJCAI, WSDM, EACL, CIKM, TPAMI, TKDE and TOIS. He also served as Area Chairs, Senior Program Committees, Editorial Board Members, and Reviewers for various academic conferences and journals.
Title: Effective and Efficient Prompt Tuning for LLM Adaptation
Abstract: With the continuous growth of large language models, the process of fine-tuning these models for new tasks has become increasingly parameter-intensive. Prompt tuning, a method that involves tuning a small set of soft prompts, has emerged as an effective and efficient approach for adapting large pre-trained language models. However, most existing prompt tuning approaches only introduce prompts at the input layer, limiting their performance and leaving large rooms for improvement. In this talk, I'll present several works that aim for efficient and effective prompt tuning of pre-trained language models. We demonstrate that existing prompt tuning can be considered as a special case of attention prompt tuning. Experimental results on the both NLP and Vision benchmark consistently demonstrate that our proposed approach outperforms state-of-the-art baselines and full fine-tuning method with pretrained models at different scales. In addition, a comprehensive set of ablation studies validate the effectiveness of the prompt design, as well as the efficiency of our approach.
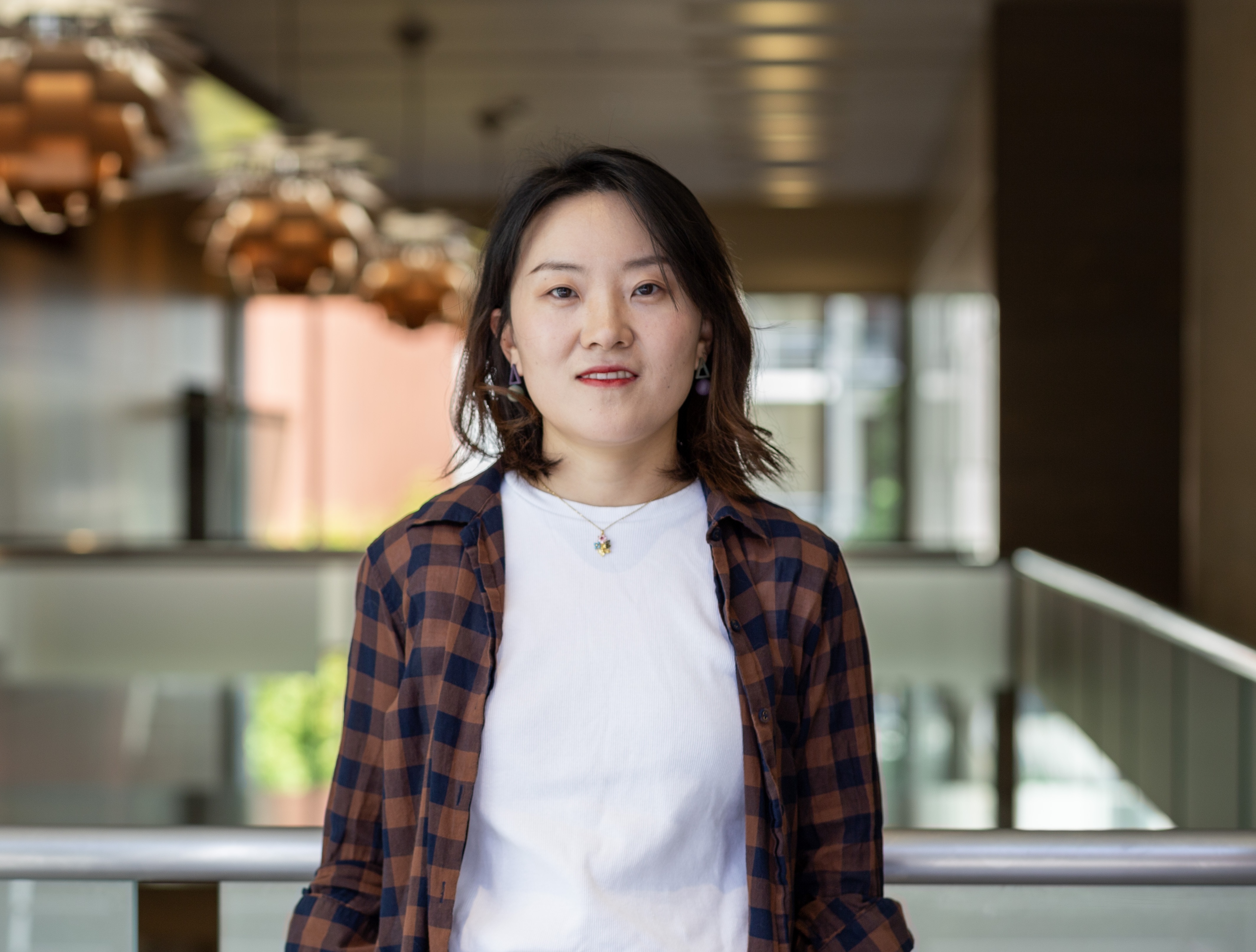
Jieyu Zhao
University of Southern California
Time: 04/16/2025, 4:30 - 5:30 PM (EST)
Place: Zoom
Bio: Jieyu Zhao is an Assistant Professor of Computer Science Department at University of Southern California. Prior to that, she was an NSF Computing Innovation Fellow at University of Maryland, College Park, working with Prof. Hal Daumé III. Jieyu received her Ph.D. from Computer Science Department, UCLA, where she was advised by Prof. Kai-Wei Chang. Her research interest lies in fairness of ML/NLP models. Her paper got the EMNLP Best Long Paper Award (2017). She was one of the recipients of 2020 Microsoft PhD Fellowship and has been selected to participate in 2021 Rising Stars in EECS workshop. Her research has been covered by news media such as Wired, The Daily Mail and so on. She was invited by UN-WOMEN Beijing on a panel discussion about gender equality and social responsibility.
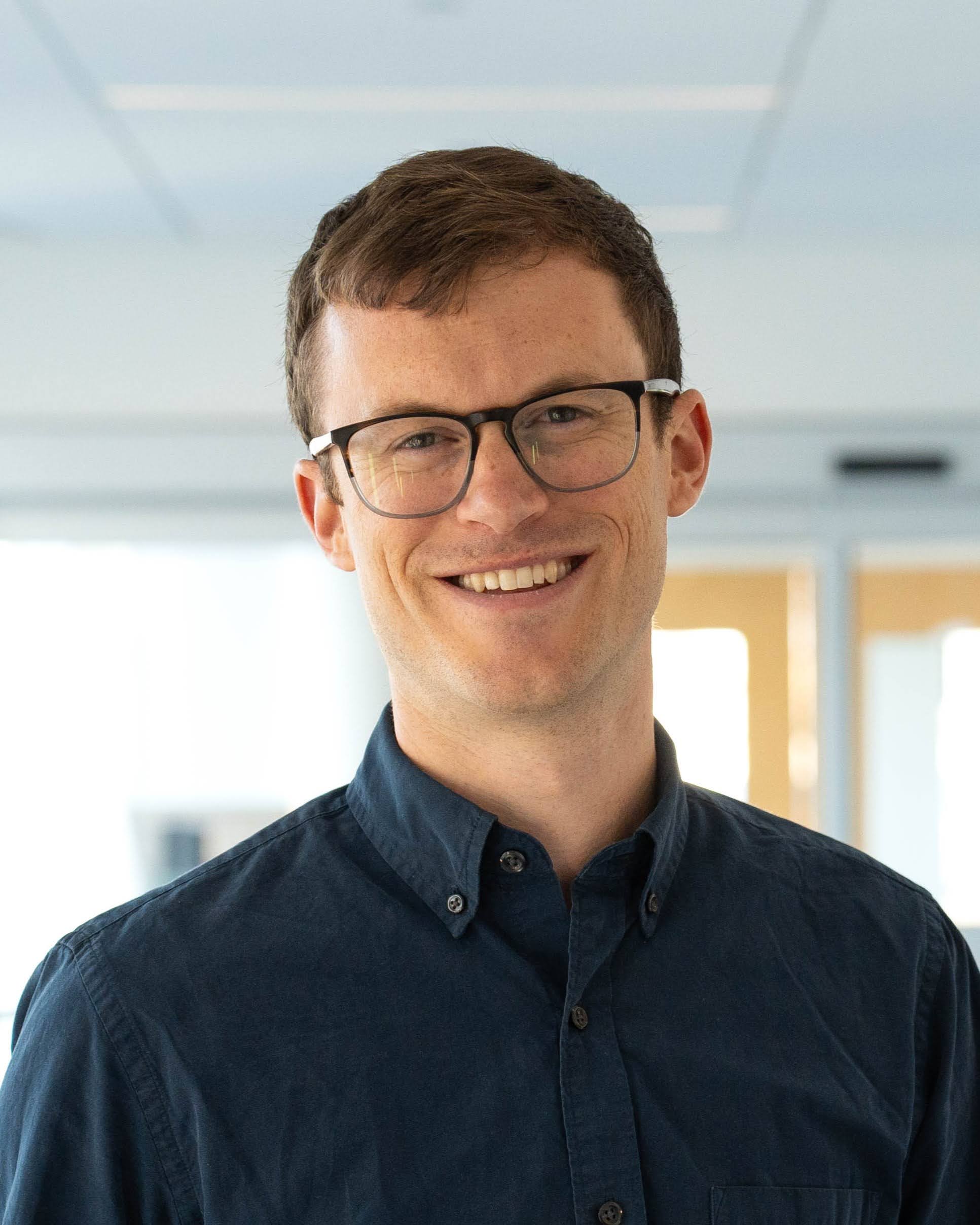
Michael Miller Yoder
University of Pittsburgh
Time: 04/23/2025, 4:30 - 5:30 PM (EST)
Place: In-person
Bio: Michael Miller Yoder is a Teaching Assistant Professor in the School of Computing and Information at the University of Pittsburgh. His teaching and research focus on data science, natural language processing, and computational social science. His work generally applies computational text analysis and other quantitative approaches to study social interaction, including how identities and ideologies are expressed. He is also interested in the social and ethical implications of data science and computational technologies.
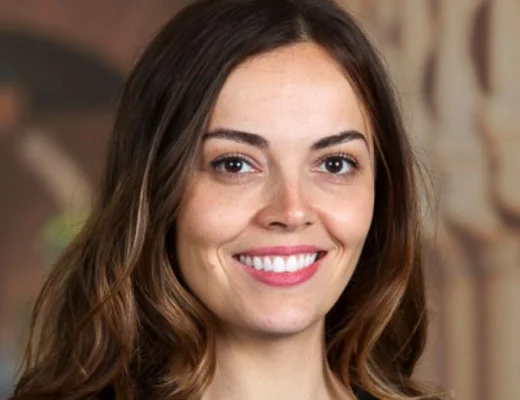
Raquel Coelho
University of Pittsburgh
Time: 09/25/2024, 15:00PM EST - 16:00PM EST
Place: In-person (Location TBD)
Bio: Rachel Coelho is jointly appointed as a Research Scientist at the Learning Research and Development Center. She holds a PhD in Learning Sciences and Technology Design combined with Education Data Science from Stanford University. Her research, rooted in sociocultural theories of learning, examines novel applications of text analytics and text generation technologies in educational contexts.
Title: Conversational Dynamics with Large Language Model-powered Chatbots: Unknown Unknowns, Suspended Conclusions, and Benefits
Abstract: This study focuses on learner reports about learning conversations to understand differences in conversation dynamics between LLM chatbots and familiar human interactions. Sociocultural theory argues talk-in-interaction between humans facilitates knowledge construction. LLM-powered chatbots are hypothesized to enhance learner meaning-making and knowledge construction. For working on lab session multiple-choice tests (MCTs), 96 students were assigned to one of three conditions: no support, peer support, or ChatGPT support. After five MCTs, they self-selected into a different group for the remaining MCTs. We focus on reflections of 11 students from follow-up semi-structured interviews who experienced both ChatGPT and peer support. Three key themes emerged. First, students observed a Problem Detection Gap: that ChatGPT cannot detect misunderstandings unless explicitly told, unlike peers or teachers. Humans engage in mutual elaboration using talk and their environment to convey understanding and identify issues. Second was the theme of Premature conclusions as students observed that AI lacks self-doubt capacity, whereas human expressions of uncertainty are crucial for learning and collaborative inquiry. For the third theme, Thinking partner with accountability, relational, and distributed expertise benefits, students highlighted social belonging, accountability, and the benefits of distributed intelligence when working with human peers rather than AI. The findings can inform strategies for deploying LLM-chatbots in education, with hopes of preserving the uniquely human aspects of learning conversations and enlightening how LLM-chatbots could augment and not replace these interactions.
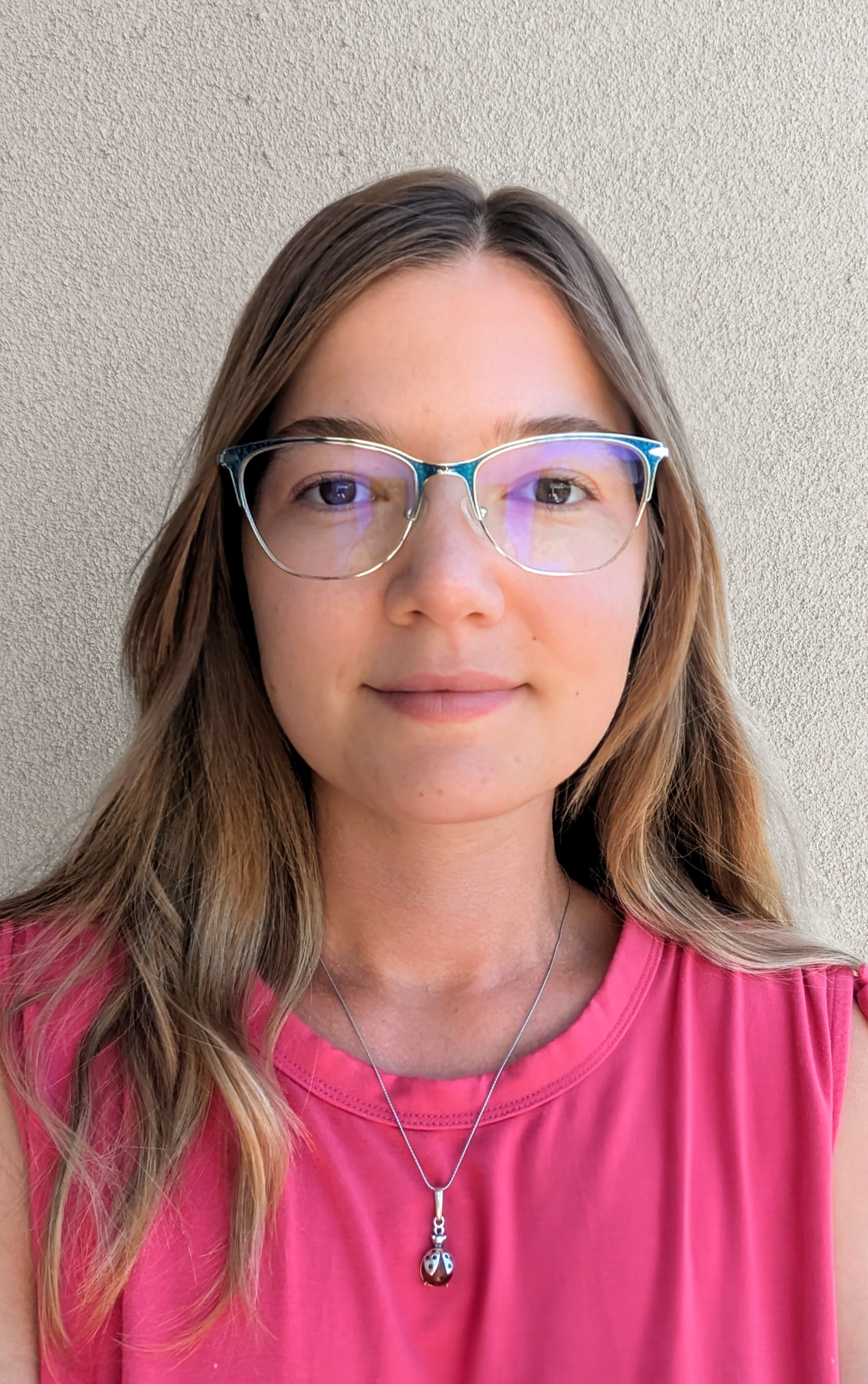
Oana Ignat (Zoom)
Santa Clara University
Time: 10/16/2024, 15:00PM EST - 16:00PM EST
Place: Zoom
Bio: Dr. Oana Ignat is an Assistant Professor in the Computer Science and Engineering (CSE) department at Santa Clara University. She completed her Ph.D. in Computer Science at the University of Michigan. Her research interests are at the intersection of Natural Language Processing (NLP) and Computer Vision (CV), where she aims to develop equitable models that work equally well across demographics such as income, race, gender, language, and culture. She is passionate about research applications for social good impact, and the use of AI to attract and retain minorities in CS. Her work resulted in several publications in top conferences such as ACL and EMNLP. She is a co-organizer of the NLP for Positive Impact (NLP4PI) workshop at EMNLP 2024 and the SemEval 2024 Task on Emotion Recognition in Low Resource Languages. Oana is also involved in several outreach programs, including co-organizing the ACL Mentorship global panel sessions and many other events and workshops centered on improving diversity in CS.
Title: Towards Inclusive Representations in Language-Vision Models
Abstract: Solving complex real-world problems often requires AI models that can process information from multiple modalities, such as language and vision, which can align with the needs of people from diverse backgrounds. An effective AI model will not only learn how to interact with humans but also do so in a way that reflects the characteristics of those it interacts with, thereby assisting in everyday life activities and significantly improving our quality of life. In this talk, I will address the problem of inclusive representations of AI Multimodal Models. I will challenge the common belief that achieving a "general understanding" is possible solely by using English data from Western countries, and I will show how current language-vision models lead to considerable performance gaps across demographics. Finally, I will highlight insights and actionable steps to address these limitations, such as the development of affordable crowdsourced geo-diverse datasets, or flexible labels that consider the data provider.
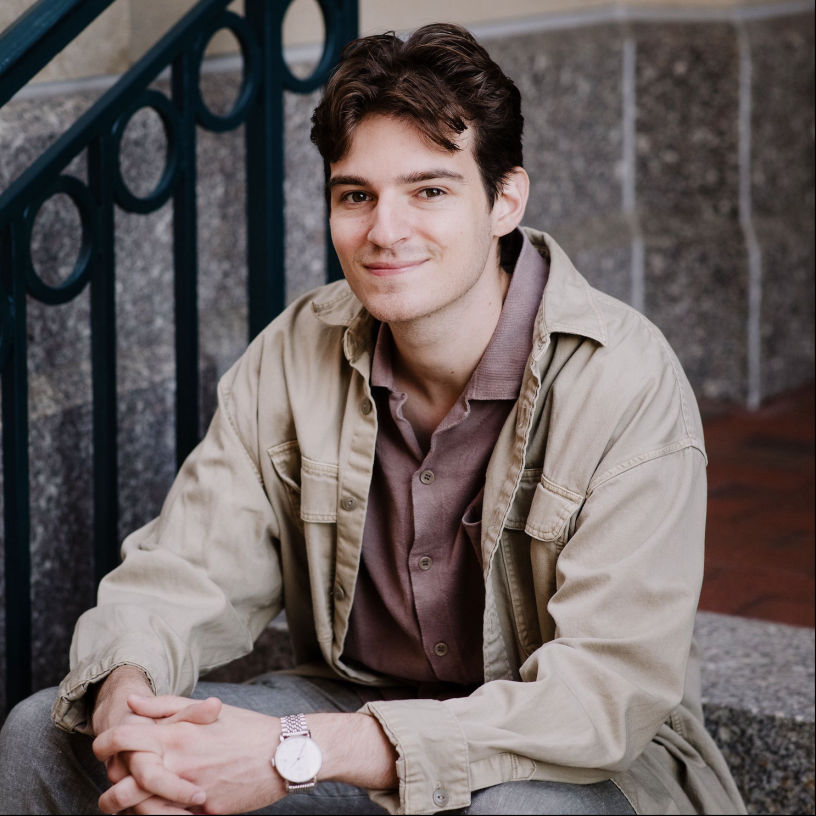
Ben Lipkin (Zoom)
Massachusetts Institute of Technology
Time: 10/23/2024, 15:00PM EST - 16:00PM EST
Place: Zoom
Bio: Ben is a 3rd year Ph.D. student in Cognitive Science at MIT, where he is advised by Roger Levy and Ev Fedorenko. His work leverages ideas from modular cognitive architecture and classical NLP/AI to implement robust, reliable, and calibrated natural language systems. He is the recipient of an NSF GRFP fellowship, an Outstanding Paper Award (EMNLP '23), and is the co-organizer of several workshops including Natural Language Reasoning & Structured Explanations (ACL '24). More information can be found on his website: https://benlipkin.github.io/
Title: Symbols and probability in the age of LLMs
Abstract: LLMs have emerged as a dominant paradigm in the design of systems that interact through text. While the capabilities of LLMs in isolation are astounding, some of the most powerful applications have come from their combination with classical symbolic infrastructure, from calculators to game engines, and probabilistic steering, from importance sampling to variational inference. In this talk, I will highlight how leveraging principles from symbolic and probabilistic computation can yield more robust and reliable systems to interact with the world of text. Across a few case studies from my work, I will present approaches that intersect language models with 1) interactive theorem provers to yield provably accurate logical reasoning, 2) probabilistic programming languages to yield uncertainty-aware semantic parsers, and 3) formal grammars to yield sampling algorithms that cannot introduce syntactic (and some semantic) errors, by design. Across these examples, I will show how these augmentations improve core aspects of performance from accuracy to calibration across an array of tasks and will present several early-stage extensions to this research space.
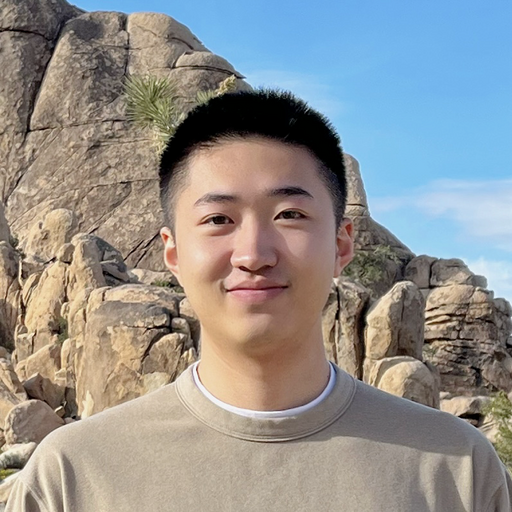
Liyan Tang (Zoom)
The University of Texas at Austin
Time: 11/06/2024, 15:00PM EST - 16:00PM EST
Place: Zoom
Bio: Liyan is a fourth-year Ph.D. student in Computer Science from the [TAUR Lab](https://taur.cs.utexas.edu/) (Text Analysis, Understanding, and Reasoning) at UT Austin advised by [Greg Durrett](https://www.cs.utexas.edu/~gdurrett/). He has been fortunate to work with [Ying Ding](https://yingding.ischool.utexas.edu/) from UT iSchool, [Yifan Peng](https://pengyifan.com/) from Weill Cornell Medicine and [Justin F. Rousseau](https://dellmed.utexas.edu/directory/justin-rousseau) from UT Southwestern Medical Center (alphabetical order). His research focuses on text generation and evaluation (especially on hallucination evaluation), as well as their applications in the clinical domain.
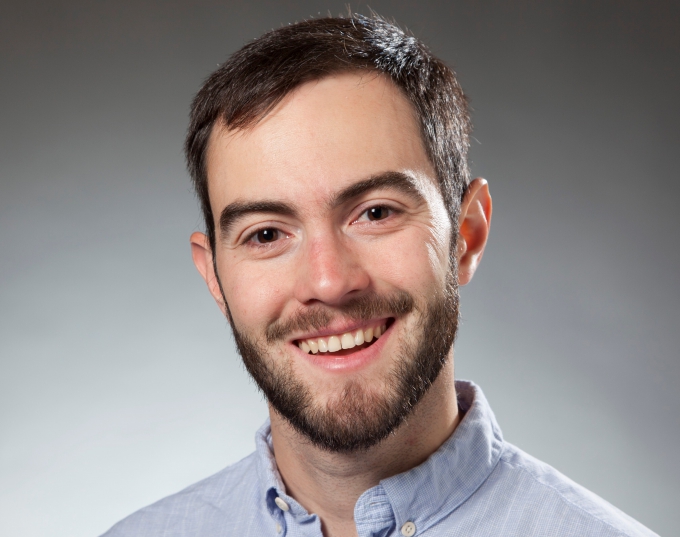
Dan Villarreal
University of Pittsburgh
Time: 11/20/2024, 15:00PM EST - 16:00PM EST
Place: In-person
Bio:
Dan Villarreal is a computational sociolinguist, as his scholarly work sits at the nexus of two research traditions: bringing together computational methods and sociolinguistic perspectives. In particular, his research seeks to expand sociolinguists' research toolkits by making computational techniques and sociolinguistic data accessible and usable; explore how speakers and listeners make sense of the tremendous phonetic variability that characterizes everyday speech; and foster a computational sociolinguistics (and a linguistics more broadly) that addresses its research questions faster, better, and more equitably. His recent work has investigated computational methods to automatically code sociophonetic variation (and how to make these methods equitable), gender segregation and speech communities in New Zealand, and whether Open Methods in linguistics contribute to academic colonialism. His research has been published in Language Variation and Change, Laboratory Phonology, and Linguistics Vanguard. Dan pronounces his last name /ˌvɪləɹiˈæl/.Title: Corpus sociolinguistics and methodological trade-offs
Abstract: The troves of speech data that have driven the increasing orientation toward large-scale methods in sociolinguistics and adjacent subfields have been, for the most part, available only to closed teams of researchers and their collaborators. This trend is beginning to change toward open data resources (e.g., Kendall & Farrington 2023; Stanford 2020). However, these resources tend to be narrowly tailored to specific research questions and/or require substantial additional annotation before researchers can realistically address sociolinguistic research questions. In this talk, I introduce a soon-to-be released open data resource—the Archive of Pittsburgh Language and Speech (APLS)—and discuss methodological trade-offs in the process of creating the corpus. As of the time of writing, APLS contains over 32 hours of audio of conversational speech from 34 Pittsburgh English speakers, consisting of over 386,000 word tokens and 900,000 force-aligned segments. (When complete, APLS will include 45 hours of audio from 40 speakers.) Powered by the corpus management software LaBB-CAT (Fromont & Hay 2012), APLS organizes linguistic data into annotation layers that are time-synchronized to speech, from the level of individual speech sounds that may be as short as 30 milliseconds, all the way to the level of entire hourlong interviews. I discuss how the methods for creating the corpus have evolved over the course of the APLS project, such as the introduction of ASR tools for transcription.
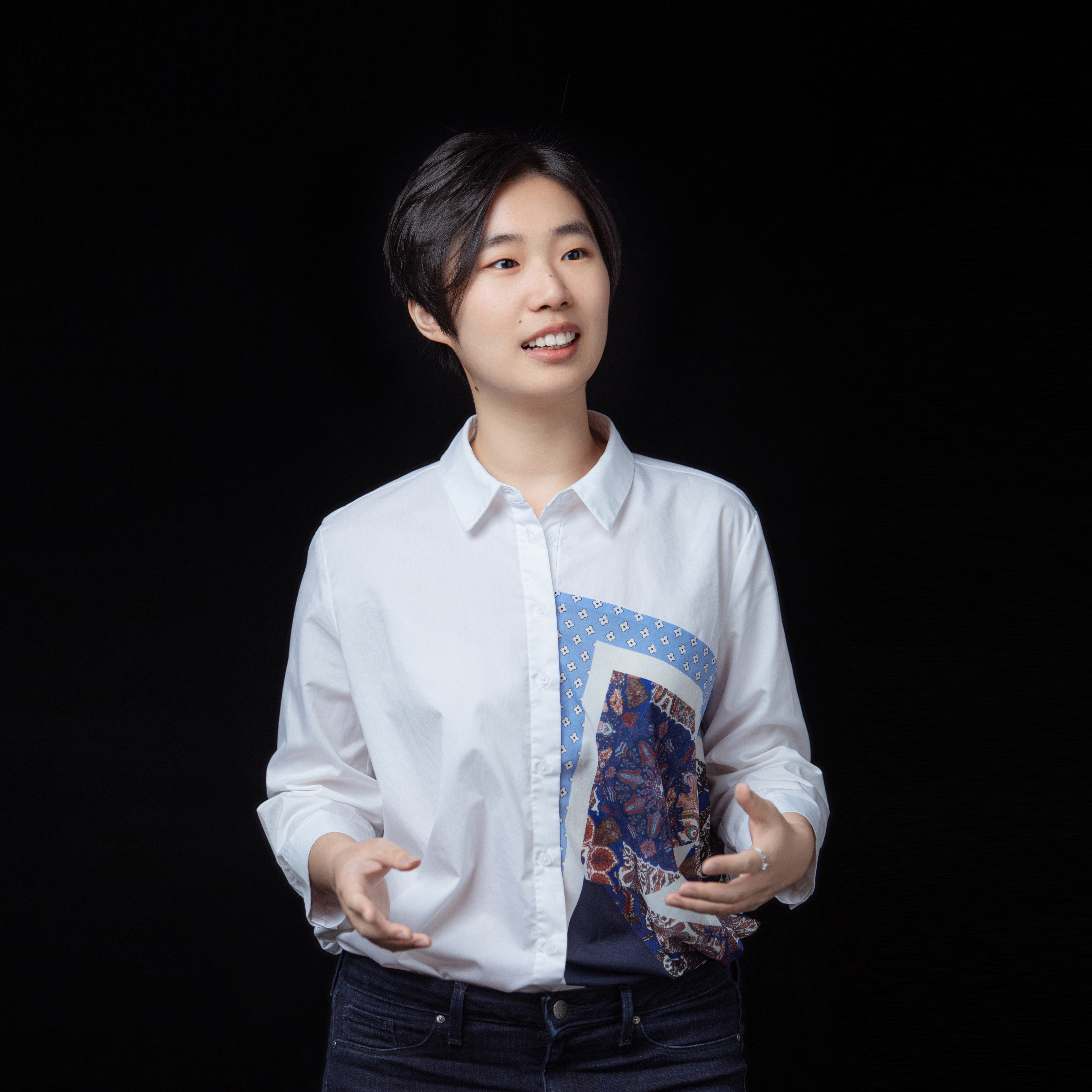
Zhijing Jin (Remote) [Recording]
Max Planck Institute & ETH
Time: 2/15/2024, 16:00am EST - 17:00am EST
Topic: Causal Inference in NLP
Title: Causal Inference for Robust, Reliable, and Responsible NLP
Abstract: Despite the remarkable progress in large language models (LLMs), it is well-known that natural language processing (NLP) models tend to fit for spurious correlations, which can lead to unstable behavior under domain shifts or adversarial attacks. In my research, I develop a causal framework for robust and fair NLP, which investigates the alignment of the causality of human decision-making and model decision-making mechanisms. Under this framework, I develop a suite of stress tests for NLP models across various tasks, such as text classification, natural language inference, and math reasoning; and I propose to enhance robustness by aligning model learning direction with the underlying data generating direction. Using this causal inference framework, I also test the validity of causal and logical reasoning in models, with implications for fighting misinformation, and also extend the impact of NLP by applying it to analyze the causality behind social phenomena important for our society, such as causal analysis of policies, and measuring gender bias in our society. Together, I develop a roadmap towards socially responsible NLP by ensuring the reliability of models, and broadcasting its impact to various social applications.
Bio: Zhijing Jin (she/her) is a Ph.D. candidate at Max Planck Institute & ETH. Her research focuses on socially responsible NLP by causal inference. Specifically, she works on expanding the impact of NLP by promoting NLP for social good, and developing CausalNLP to improve robustness, fairness, and interpretability of NLP models, as well as analyze the causes of social problems. She has published at many NLP and AI venues (e.g., ACL, EMNLP, NAACL, NeurIPS, AAAI, AISTATS). Her work has been featured in MIT News, ACM TechNews, and Synced. She is actively involved in AI for social good, as the co-organizer of three NLP for Positive Impact Workshops (at ACL 2021, EMNLP 2022, and EMNLP 2024), Moral AI Workshop at NeurIPS 2023, and RobustML Workshop at ICLR 2021. To support the NLP research community, she organizes the ACL Year-Round Mentorship Program. To foster the causality research community, she organized the Tutorial on CausalNLP at EMNLP 2022, and served as the Publications Chair for the 1st conference on Causal Learning and Reasoning (CLeaR). More information can be found on her personal website: zhijing-jin.com
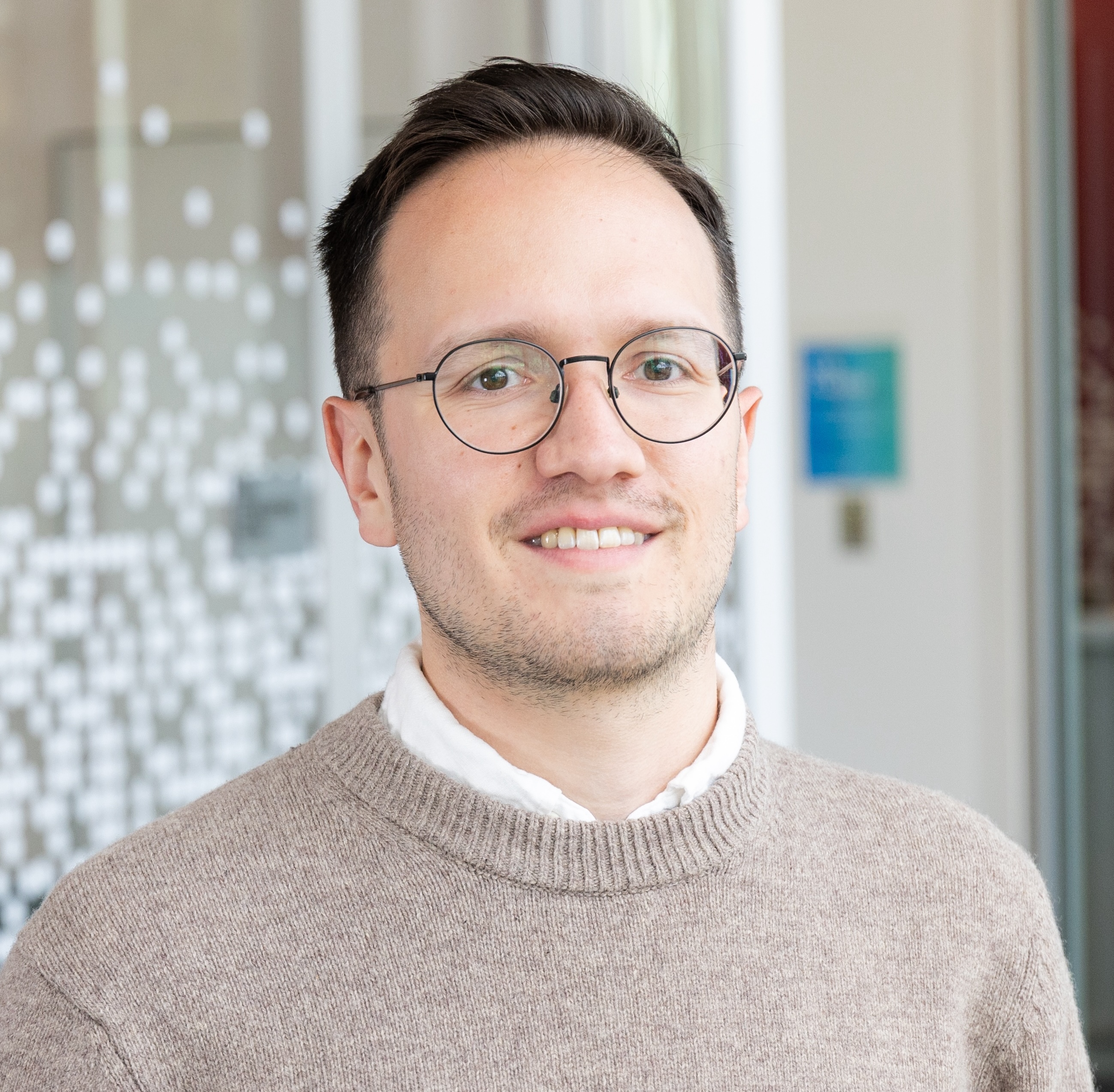
Aaron Mueller (Remote)
Khoury College of Computer Sciences, Northeastern University
Time: 2/29/2024, 16:00am EST - 17:00am EST
Place: Zoom and 5th floor in 130 N Bellefield Ave
Title: Evaluating and Surgically Improving Generalization in Language Models
Abstract: As language models (LMs) are deployed in wider applications, understanding and controlling how they generalize becomes increasingly important. However, it is difficult to directly evaluate how models are accomplishing the tasks we give them—and when needed, it is not obvious how to improve generalization on a task without destroying general capabilities. In this talk, I will present two projects that tackle these challenges. I will first present an evaluation of how models process language structure: we evaluate out-of-domain generalization in in-context learning settings, finding that pre-training on code may result in more robust generalization. We also find that chain-of-thought (CoT) results can be misleading: CoT often only improves in-distribution performance without improving out-of-distribution performance. Then, I will present an ongoing mechanistic interpretability effort to isolate and control the algorithms LMs implement via feature circuits. By learning sparse human-interpretable encodings of models’ hidden states (features) and discovering circuits on them, we observe how LMs perform subject-verb agreement: by composing representations of grammatical number in the MLPs and residuals, while detecting and learning to ignore distractor clauses in the attention heads. I will conclude by showing an application of feature circuits—ablating spurious features to improve the generalization of a classifier.
Bio: Aaron Mueller is a Zuckerman postdoctoral fellow working with David Bau (Northeastern U.) and Yonatan Belinkov (Technion). He obtained his PhD from Johns Hopkins University supervised by Tal Linzen. His work spans topics in the intersection of natural language processing and psycholinguistics, including causal interpretability, NLP evaluations inspired by linguistic principles, and efficient language acquisition. He was an NSF Graduate Fellow, and has received an Outstanding Paper Award from ACL (2023), a Featured Paper recognition from TMLR (2023), and coverage in the New York Times as an organizer of the BabyLM Challenge.
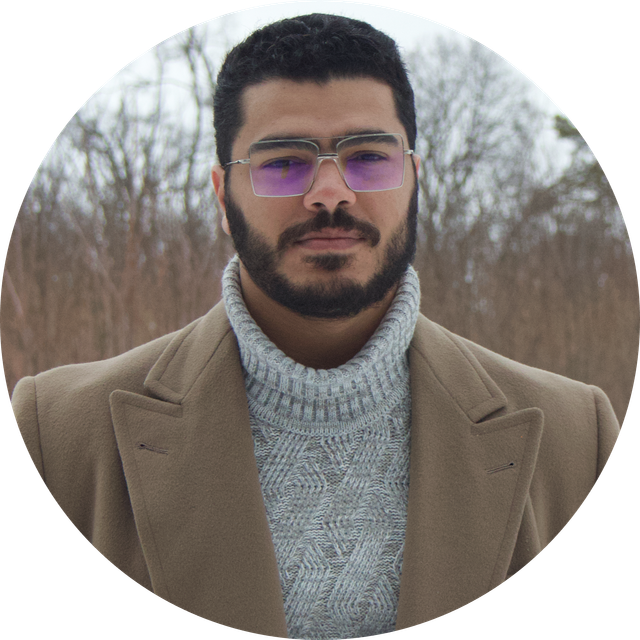
Muhammad Khalifa (Remote)
University of Michigan in Ann Arbor
Time: 3/21/2024, 16:00am EST - 17:00am EST
Place: Zoom and 5th floor in 130 N Bellefield Ave
Bio: Muhammad Khalifa is a third-year PhD candidate at the University of Michigan in Ann Arbor and an intern at Ai2. He is advised by Lu Wang and Honglak Lee. His main research interests are Large Language Models, Reasoning, and Controlled Generation. He spent 10 months at Amazon AI working with Miguel Ballesteros and Kathy Mckeown on multiple projects including Dialogue Summarization and Semi-structured documents understanding. Prior to that, hw was an intern at Naver Labs Europe where he worked on Controllable Text Generation and Energy-based models with Hady Elsahar and Marc Dymetman.
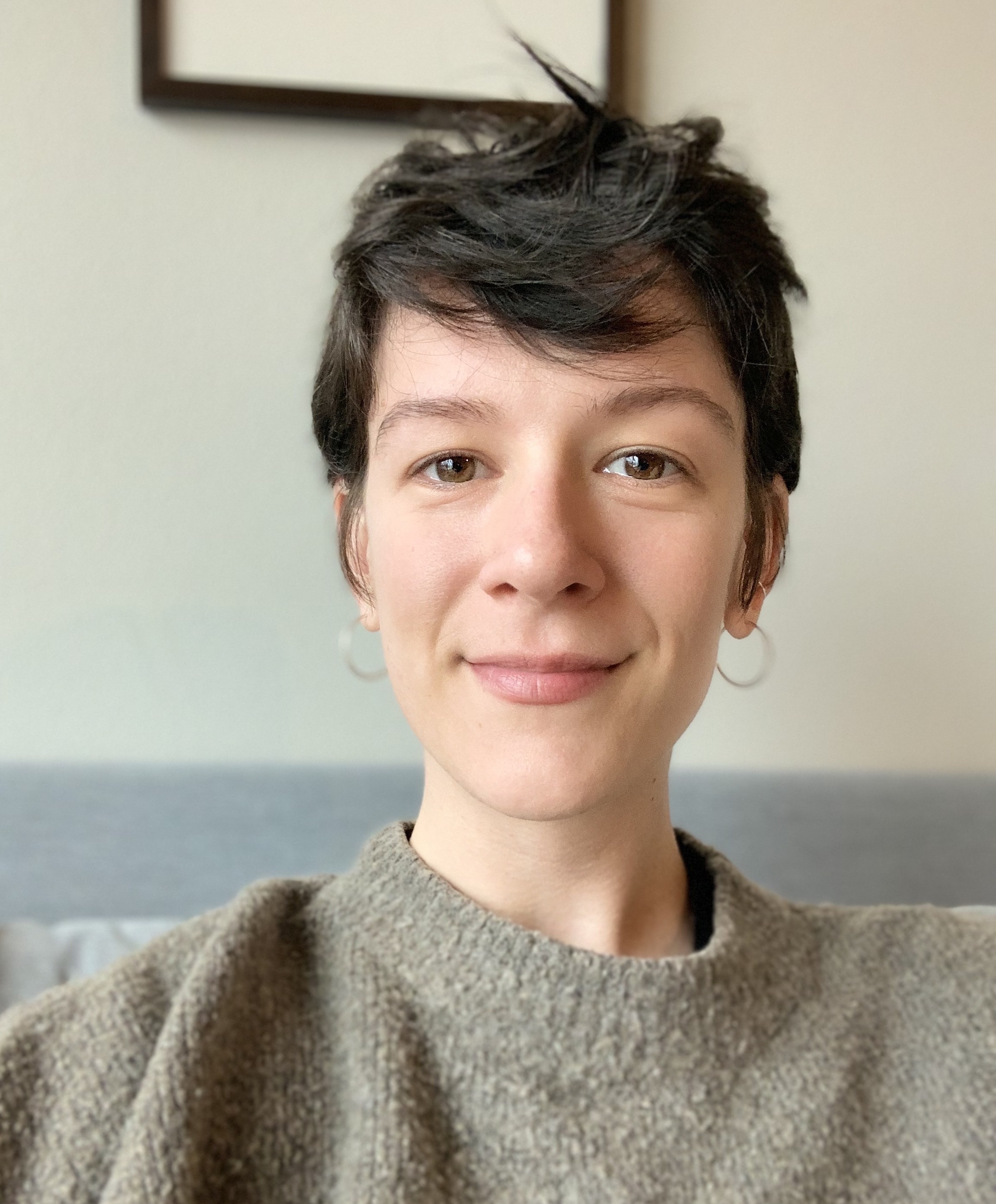
Ana Marasović (Remote)
the Kahlert School of Computing at the University of Utah
Time: 4/4/2024, 16:00am EST - 17:00am EST
Place: Zoom and 5th floor in 130 N Bellefield Ave
Bio: Ana Marasović is an Assistant Professor in the Kahlert School of Computing at the University of Utah. Her primary research interests are at the confluence of natural language processing (NLP), explainable artificial intelligence (XAI), and multimodality. She is interested in projects that (1) rigorously validate AI technologies, and (2) make human interaction with AI more intuitive. For an example of robust validation check out her work on carefully designing benchmarks to validate the robustness of QA models in the presence of common linguistic phenomena such as negation or coreference. On the other hand, to help people create a mental model about how to interact with AI, she has contributed to building models that self-explain their predictions in a way that is easily understandable to people, for example by saying why did the model give this answer instead of another one (contrastive explanations) or by telling in plain language the gist of its reasoning (free-text explanations). Moving forward, she is excited to evaluate and improve such models with application-grounded, human-subject evaluations. Previously, Ana Marasović was a Young Investigator at the Allen Institute for AI from 2019–2022 where she worked with Noah A. Smith and Yejin Choi. During that time she also had a courtesy appointment in the Paul G. Allen School of Computer Science & Engineering at the University of Washington. Ana Marasović received her Ph.D. degree at Heidelberg University, where she was advised by Anette Frank. Before receiving her PhD in 2019, she completed her B.Sc. (2013) and M.Sc. (2015) in Mathematics at the University of Zagreb.
Joel Tetreault (Remote)
Dataminr
Time: 4/11/2024, 16:00am EST - 17:00am EST
Zoom and 5th floor in 130 N Bellefield Ave
Title: A Brief History of Natural Language Processing
Abstract: The title says it all! As the field of Natural Language Processing (NLP) continues to make incredible strides and advancements, it's important to take a step back and use the past to understand the current transformations. Drawing from literature and interviews, we'll dive into the early years of NLP and explore some of the major themes, trends, and personalities that paved the way for the cutting-edge technology we have today.
Bio: Joel Tetreault is VP of Research at Dataminr, a company that provides updates on breaking events across the world in real-time. His background is in AI, specifically Natural Language Processing and Machine Learning, and using techniques from those fields to solve real-world problems such as automatic essay scoring, grammatical error correction, hate speech detection, real-time event detection, and dialogue systems, AI for Good, among others. Prior to joining Dataminr, he led research groups at Grammarly, Nuance, and Educational Testing Service, and was a Senior Research Scientist at Yahoo Labs. Joel was one of the program chairs of ACL 2020 and also one of the longest-serving members of the NAACL Board where he was Treasurer for six years. Additionally, he was a long-time organizer of the Building Educational Application workshop series (10+ years) and organized workshops on Generation, AI for Social Good, Abusive Language, Metaphor and Event Detection.
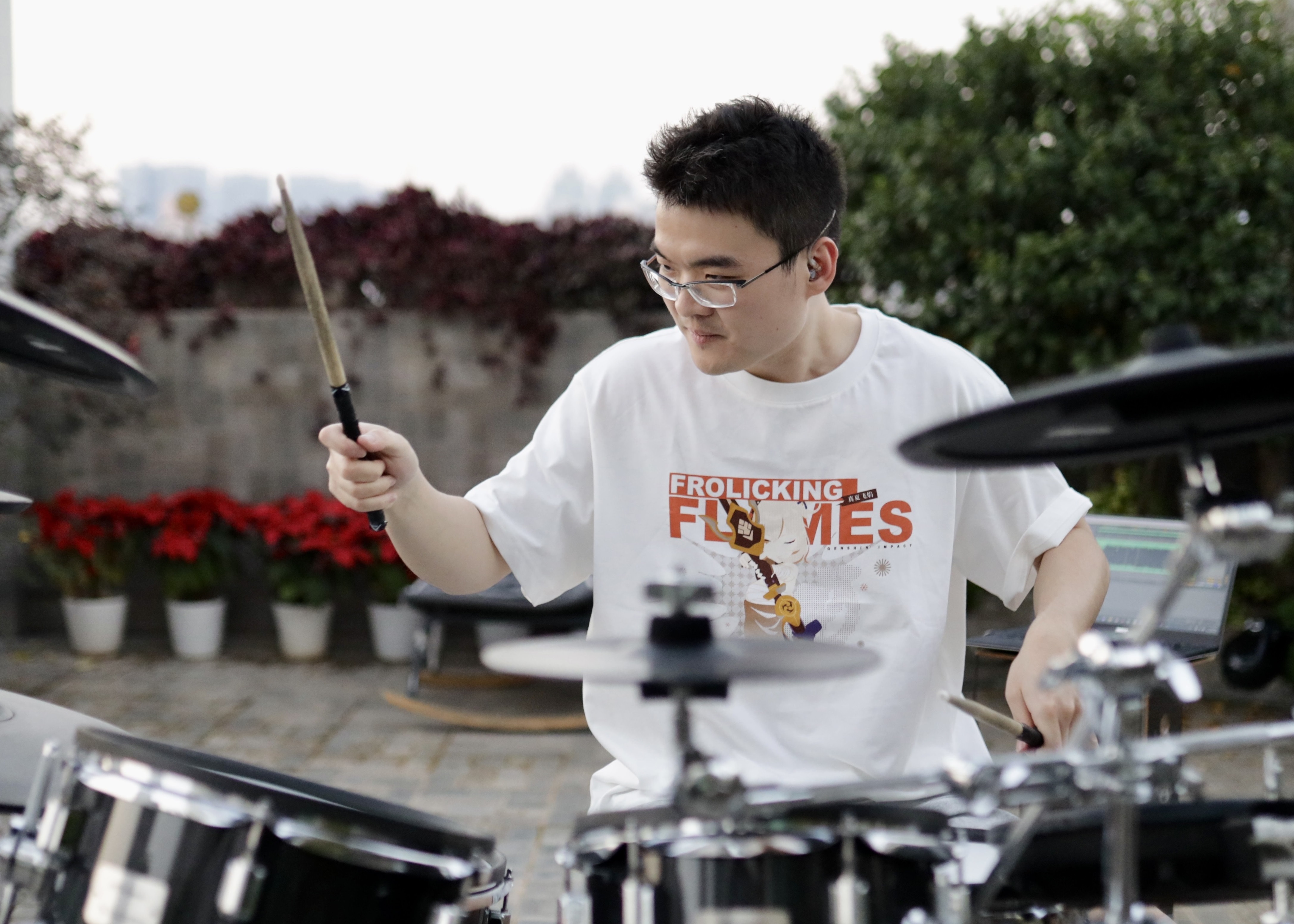
Zhang "Harry" Li (Remote)
University of Pennsylvania
Time: 4/18/2024, 16:00am EST - 17:00am EST
Zoom and 5th floor in 130 N Bellefield Ave
Title: Structured Event Reasoning with Large Language Models
Abstract: Reasoning about real-life events is a unifying challenge in AI and NLP that has profound utility in a variety of domains, while any fallacy in high-stake applications like law, medicine, and science could be catastrophic. Able to work with diverse text in these domains, large language models (LLMs) have proven capable of answering questions and solving problems. In this talk, I demonstrate that end-to-end LLMs still systematically fail on reasoning tasks of complex events. Moreover, their black-box nature gives rise to little interpretability and user control. To address these issues, I propose two general approaches to use LLMs in conjunction with a structured representation of events. The first is a language-based representation involving relations of sub-events that can be learned by LLMs via fine-tuning. The second is a symbolic representation involving states of entities that can be leveraged by either LLMs or deterministic solvers. On a suite of event reasoning tasks, I show that both approaches outperform end-to-end LLMs in terms of performance and trustworthiness.
Bio: Li "Harry" Zhang is a 5th-year PhD student working on Natural Language Processing (NLP) and artificial intelligence at the University of Pennsylvania advised by Prof. Chris Callison-Burch. He earned his Bachelor's degree at the University of Michigan mentored by Prof. Rada Mihalcea and Prof. Dragomir Radev. He has published more than 20 papers in NLP conferences that have been cited more than 1,000 times. He has reviewed more than 50 papers in those venues and has served as Session Chair and Program Chair in many conferences and workshops. Being also a musician, producer, content creator of over 50,000 subscribers, he is passionate in the research of AI music.